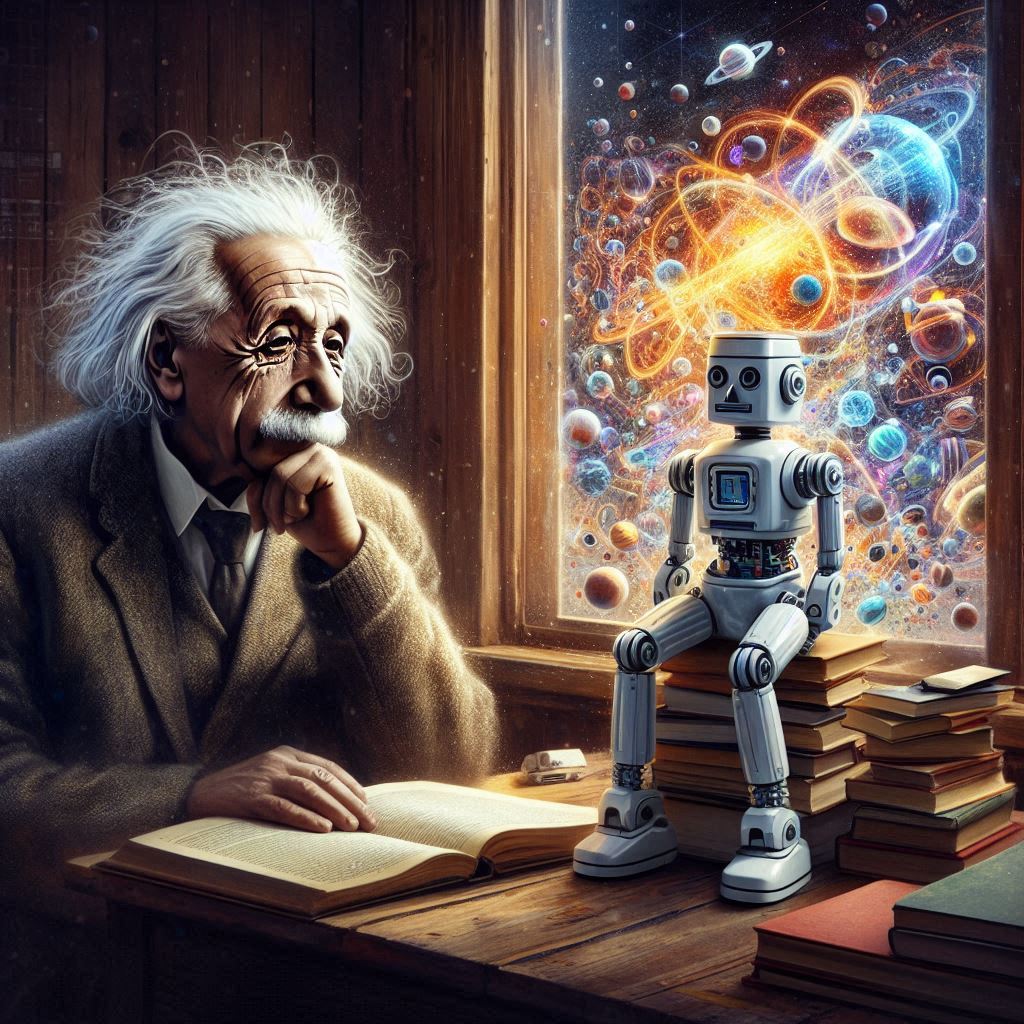
Why AI Won’t Be Einstein Anytime Soon
I keep coming back to Thomas Wolf‘s brilliant essay about AI becoming “yes-men on servers.” As someone who spends my days advising organisations on AI strategy and my evenings experimenting with these tools, his perspective cuts through the noise with refreshing clarity.
The more I use generative AI, the more I see brilliant dot-connectors rather than breakthrough innovators. They’ll surprise me by linking information from entirely different domains—connecting dots I hadn’t noticed were even on the same page—but I’ve yet to witness one create a genuinely original picture. These systems excel at remixing the familiar rather than imagining the unknown.
Not Just More Compute, Different Thinking
Wolf cuts through the scaling narrative with surgical precision. “The main mistake people usually make,” he notes, “is thinking Newton or Einstein were just scaled-up good students.” This elegant observation dismantles the entire foundation of today’s AI scaling race.
Einstein didn’t revolutionise physics by processing more information than his contemporaries. He reimagined the questions themselves. He considered what reality would look like if certain fundamental assumptions—ones so basic no one thought to question them—were wrong.
This is the chasm between human breakthrough thinking and AI’s pattern matching that no amount of training data or parameter scaling seems poised to bridge. Revolutionary thinkers don’t just connect dots more efficiently; they question why we drew those particular dots in the first place.
The Convenient Narrative of Inevitable AGI
While OpenAI’s Sam Altman speaks of “superintelligent” AI that will “massively accelerate scientific discovery” and Anthropic’s Dario Amodei envisions AI curing most cancers, Wolf offers a perspective untethered from fundraising narratives and valuation targets.
Let’s acknowledge what today’s AI systems genuinely are: remarkable achievements in pattern recognition. They’ll summarise research papers, generate passable marketing copy, and perform narrow tasks with impressive accuracy. But fundamentally, they’re sophisticated prediction engines, calculating what should come next based on what has come before. They’re backward-looking by design, which makes revolutionary forward thinking structurally problematic.
Measuring the Wrong Things Entirely
The “evaluation crisis” Wolf identifies might be my favourite part of his argument. Our AI benchmarks reward systems for correctly answering questions with known answers. But genuine breakthroughs rarely come from answering existing questions correctly—they emerge from asking questions no one thought to ask.
When was the last time a scientific revolution began with, “Let me solve this well-defined problem with a clear answer”? Einstein didn’t set out to improve the accuracy of Newtonian physics by 3.7%. He questioned whether our entire conception of space and time was fundamentally flawed.
Follow the Money (and the Computing Contracts)
I find it telling that the loudest voices predicting imminent artificial general intelligence (AGI) often have direct financial interests in that narrative. Without casting aspersions on individual motivations, it’s worth noting the correlation between AGI prediction timelines and funding cycles.
The current paradigm of throwing more computing power at statistical pattern matching seems unlikely to yield systems capable of the creative leaps that define breakthrough human intelligence. But claiming otherwise does drive investment, cloud computing contracts, and media attention.
The Critical Space Between Hype and Disappointment
What fascinates me about Wolf’s perspective is how it carves out a nuanced middle ground in the AI conversation. We’re stuck in a peculiar moment where AI simultaneously does more than many expected (“it wrote this email as if it were me!”) and fundamentally less than the visionaries promise (“it will solve climate change by next Tuesday”).
Business leaders navigating this landscape face a genuine challenge. They’re bombarded with contradictory messages: consultants selling AI as existential transformation, critics dismissing it as glorified autocomplete, and vendors promising their particular flavour of AI will revolutionise everything from customer service to product development.
The truth, as ever, lies in the messy middle.
The most successful organisations I’ve advised aren’t asking “How do we replace humans with AI?” They’re exploring “How does AI change the questions humans should be asking?” They understand that the technology’s real power isn’t in automating creativity but in clearing the decks so humans can focus on more creative endeavours.
The Perspective Shift That Matters
Wolf’s closing observation strikes at the heart of what makes this conversation important: “We don’t need an A+ student who can answer every question with general knowledge. We need a B student who sees and questions what everyone else missed.”
This isn’t just about technological capabilities—it’s about how we evaluate intelligence itself. Our fixation on perfect recall and flawless execution misses the essential unpredictability of genuine innovation.
So perhaps instead of waiting for AI to deliver Einstein-level breakthroughs, we should focus on how it frees humans to ask better questions. After all, connecting the dots differently is valuable, but questioning why we’re connecting these particular dots in the first place? That remains remarkably, stubbornly human.
What’s your experience with generative AI? Have you seen it create truly new insights, or primarily connect existing information in valuable but ultimately derivative ways? I’d be interested to hear your thoughts.
#ArtificialIntelligence #FutureOfAI #AIStrategy #TechInnovation #BusinessLeadership
Ger Perdisatt is Founder & CEO of Acuity AI, providing independent AI advisory services to organisations navigating the complex landscape of artificial intelligence adoption.